Knowledge Graphs and Natural Language Processing. The Year of the Graph Newsletter Vol. 15, July/August 2019
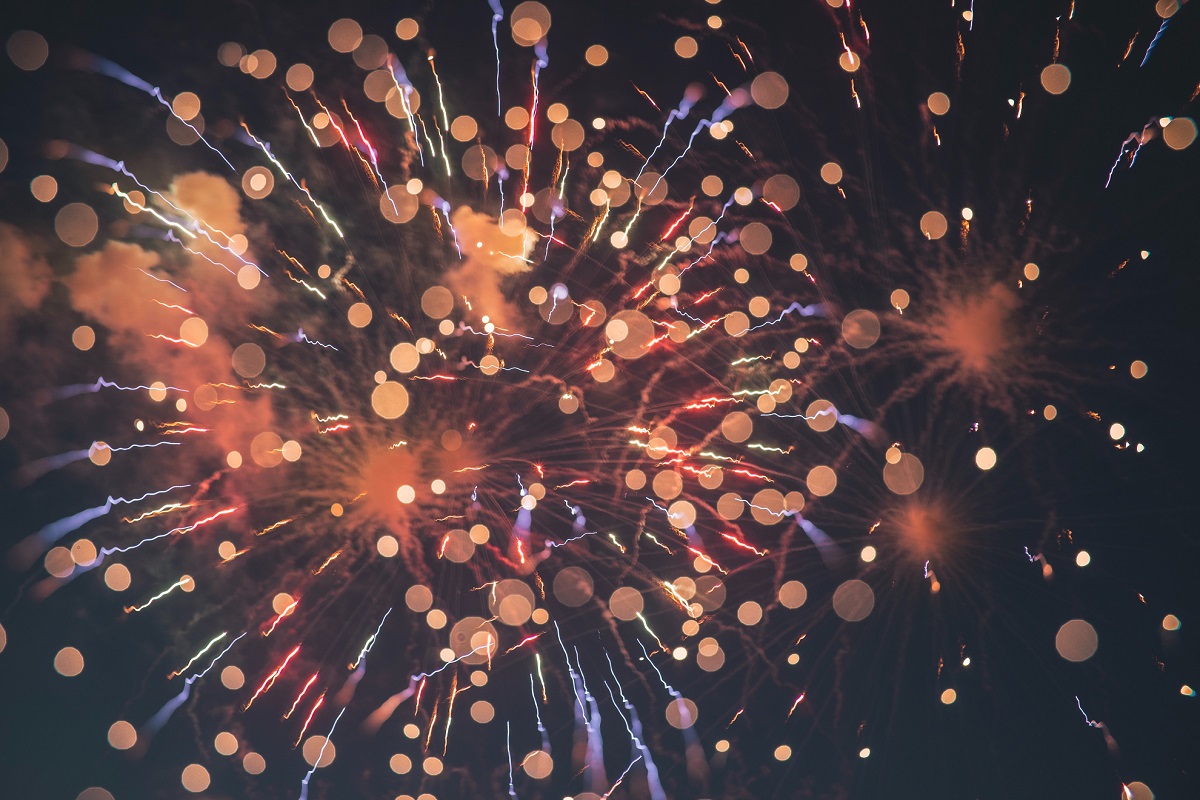
Pinterest gets with the knowledge graph program. Facebook releases a new dataset for conversational Reasoning over Knowledge Graphs. Connected Data London announces its own program, rich in leaders and innovators.
And as always, new knowledge graph and graph database releases, research, use cases, and definitions. A double bill summertime newsletter edition, making your knowledge graph living easy.
Pinterest adopted Semantic Web technologies to create a knowledge graph that aims to represent the vast amount of content and users, to help both content recommendation and ads targeting. A mixed team from Stanford and Pinterest present the engineering of an OWL ontology—the Pinterest Taxonomy—that forms the core of Pinterest’s knowledge graph, the Pinterest Taste Graph.
Use of OWL and Semantic Web Technologies at Pinterest
Pinterest started out editing the Pinterest Taxonomy in spreadsheets. Soon, the Pinterest Content team realized that it was difficult to visualize, keep track of changes, and associate interests with metadata. Thus, Pinterest decided to adopt a standard knowledge representation language and more appropriate tooling for collaborative taxonomy editing.
Facebook AI researchers study a conversational reasoning model that strategically traverses through a large-scale common fact knowledge graph to introduce engaging and contextually diverse entities and attributes in conversational agents. As part of this, they created a corpus called OpenDialKG.
OpenDialKG was created using the Freebase knowledge graphs and 15K conversations between human agents. The conversations were recorded, referenced Freebase entities were identified, and the paths corresponding to the reference of entities in the flow of the conversation added to OpenDialKG.
The DialKG Walker model was developed, that learns the symbolic transitions of dialog contexts as structured traversals over KG, and predicts natural entities to introduce given previous dialog contexts via a novel domain-agnostic, attention-based graph path decoder.
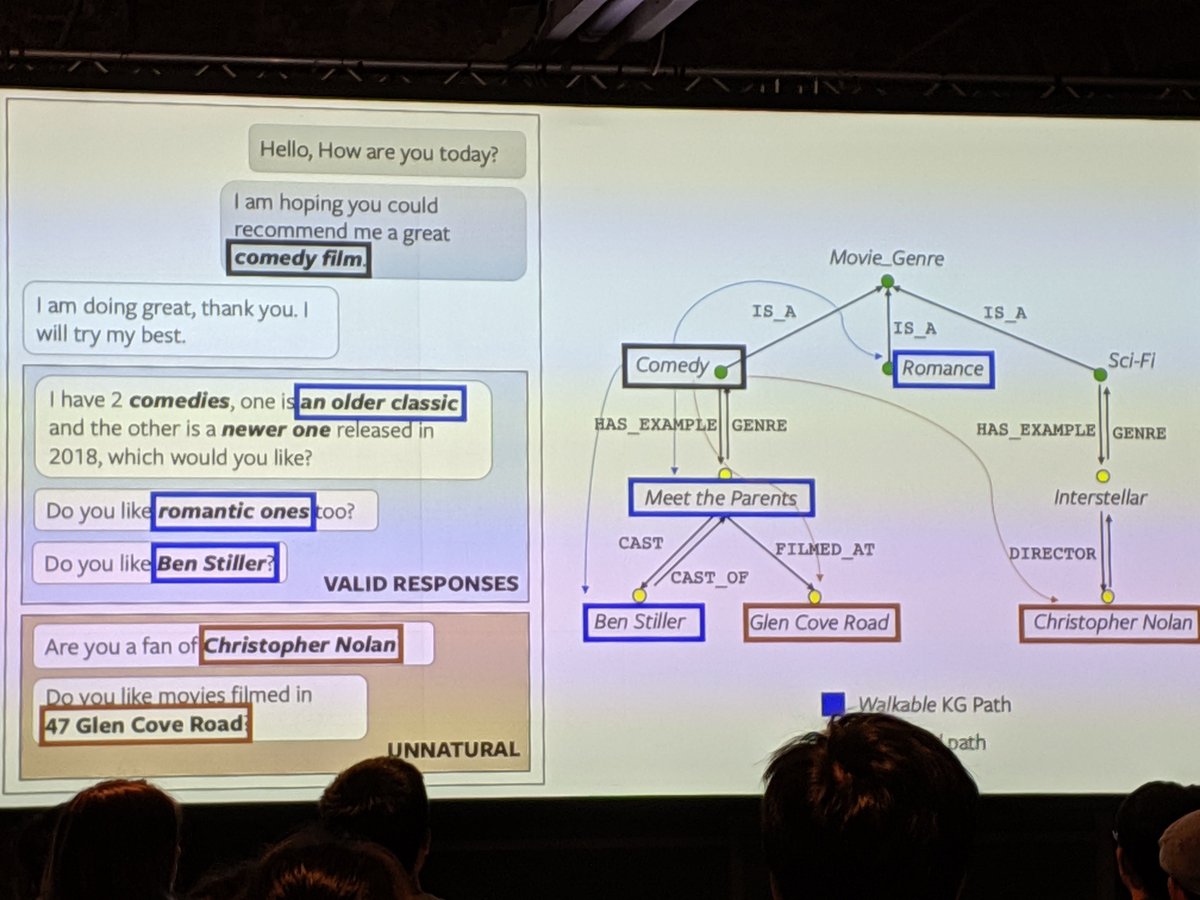
OpenDialKG: Explainable Conversational Reasoning with Attention-based Walks over Knowledge Graphs
Automatic and human evaluations show that our model can retrieve more natural and human-like responses than the state-ofthe-art baselines or rule-based models, in both in-domain and cross-domain tasks. The proposed model also generates a KG walk path for each entity retrieved, providing a natural way to explain conversational reasoning.
The OpenDialKG research was published in ACL 2019, the annual meeting of the Association for Computational Linguistics. ACL 2019 was enormous — 2900 submissions, 660 accepted papers, more than 3000 registered attendees, and four workshops with about 400 attendees. About 30 papers out of 660, or 5%, involved Knowledge Graphs.
Michael Galkin from Fraunhofer IAIS outlined some major areas where KGs were most represented and described some very promising papers. Galkin notes research in Dialogue Systems over Knowledge Graphs. Natural Language Generation of Knowledge Graph facts. Complex Question Answering over Knowledge Graphs. Named Entity Recognition and Relation Linking over KGs. KG Embeddings & Graph Representations.
Bonus track: natural language interfaces for databases, including both relational databases and RDF graph databases

Knowledge Graphs in Natural Language Processing @ ACL 2019
It gets visible that both goal-oriented and chit-chat agents have to possess some knowledge, i.e., domain knowledge for goal-oriented agents and more of commonsense knowledge for chit-chat agents. This can’t be expressed clearer than by the ACL president Ming Zhou himself — in his welcome speech he emphasized the importance of incorporating knowledge graphs, reasoning, and context into dialogue systems.
Connected Data London, the leading event for those who use the relationships, meaning and context in Data to achieve great things, has announced its lineup for 2019. The biggest and most visionary event to feature the rich array of technologies which make up the Connected Data landscape is taking place in London on October 3 and 4, 2019.
Building on a tradition dating back long before analysts like Gartner proclaimed knowledge graphs a key technology of the 2020s, the conference focuses on Knowledge Graphs and Graph Databases, AI and Machine Learning, Linked Data and Semantic Technology.

Connected Data London 2019 program announced: The Future is Graph – Knowledge Graph. Connected Data London is where it unfolds
Keynote presentations by Uber’s Joshua Shinavier, and Microsoft’s David Gorena will explore the present and future of knowledge graphs at scale, from philosophy and cognition in Search of the Universal Data Model, to AI applications in the cloud powered by the Largest Graph of Human Activity at Work ever Created. The event also features leading experts and practitioners from the likes of Bayer, GSK, JP Morgan, Farfetch, Inrupt, nVidia, Refinitiv, and Siren.io.
What is a knowledge graph? Mike Bergman from Cognonto Corp. embarks on a journey through various knowledge graph definitions found in literature. Bergman notes that if one looks up what is a knowledge graph, one finds there are some 99 references on Google, plus 22 academic papers. Bergman presents and compares various definitions of knowledge graph.

A Common Sense View of Knowledge Graphs
I present and compare various definitions of knowledge graph. However, to start, from a common-sense standpoint, we can easily decompose an understanding of the phrase from its composite terms. Knowledge seems straightforward, but an inspection of the literature and philosophers over time indicates the concept is neither uniformly understood nor agreed
Alan Morrison from PwC goes one step further, elaborating on the difference between knowledge graphs and a graph databases. You would think this should be clear, but we’ve seen a good deal of confusion, ranging from users to “influencers”. The clarity Morrison offers is much needed.
What is the difference between a knowledge graph and a graph database?
A knowledge graph is a knowledge base that’s made machine readable with the help of logically consistent, linked graphs that together constitute an interrelated group of facts. Graph databases are often used to store knowledge graph data
Graph databases have a lot of hype right now, but as DataStax’s Dave Bechberger puts it, this does not mean there isn’t real value there. But there’s a number of things you need to do to cut through the hype. Bechberger shared his experience, Kurt Cagle weighs in his Forbes article, and a number of experts share their opinions to provide a well-rounded view.

Why Experts See Graph Databases Headed for Mainstream Use
Graph databases are now clearly riding the upward trend toward mainstream adoption for which the sector has been waiting for several years. To get a snapshot on current thinking about graph’s future, seven industry experts were asked the following question: “Graph Is dominating the database market; where will it be in 2022?”
Graph database Stardog announced its latest version 7, featuring up to 20x write performance improvements, knowledge graph virtual transparency, and schema multi-tenancy.
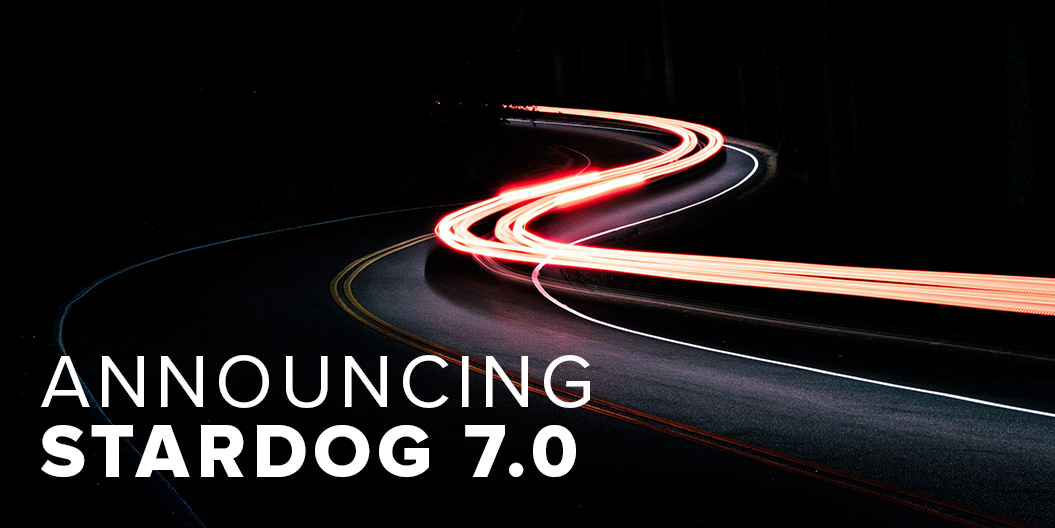
Announcing Stardog 7
Stardog 7 is dramatically faster, typically between 10x and 20x improvement for writes. We think Stardog’s unique combination of graph, storage, and virtualization is a data management game changer. Apps can share and reuse connected data hosted in Stardog 7 without stepping on each other’s toes or, just as crucially, without requiring a single schema to rule all the others
Another graph database, Dgraph, lead by ex-Googler Manish Jain, announced its $11,5M Round A funding to pursue its unique and opinionated path.

Graph database reinvented: Dgraph secures $11.5M to pursue its unique and opinionated path
Imagine a graph database that’s not aimed at the growing graph database market, selling to Fortune 500 without sales, and claiming to be the fastest without benchmarks. Dgraph is unique in some interesting ways
Wikidata has grown to become one of the most important knowledge graphs in the world. But what is it exactly? Lydia Pintscher is Wikidata’s Product Manager, and here she goes back to Wikidata’s origins, and explains in simple terms what it is, how it works, how it’s connected with Wikipedia and what its benefits are.
In addition, a group of researchers from Society Byte conducted a research on Wikidata in order to estimate class completeness in its collaborative knowledge graph environment. They experimentally evaluated their methods in the context of Wikidata, concluding that their convergence metric can be leveraged to identify gaps in the knowledge graph.

Wikidata – Wikipedia’s not so little sister is finding its own way
Making Wikipedia semantic is making its content accessible to machines to enable answering questions based on the content, and make the content easier to reuse and remix. Wikidata should be a central place that stores general purpose data (like those found in those “infoboxes” on Wikipedia) related to the millions of concepts covered in Wikipedia articles
A new public knowledge graph is born. TheyBuyForYou is a three-year initiative bringing together researchers, innovators and public administrations from 5 European countries. Its aim is to make procurement data more easily accessible and hence facilitate better decisions in areas such as economic development, demand management, competitive markets and vendor intelligence.
To achieve this, TheyBuyForYou leverages a range of data sources which are integrated into a knowledge graph, which is used in data analytics and decision making. The team announced the first release of the TheyBuyForYou knowledge graph, integrating tender and company data. As of the first quarter of 2019, the graph consists of over 23 million triples (records), covering information about almost 220,000 tenders.

Enabling procurement data value chains through a knowledge graph-based platform
Public procurement affects organisations across all sectors. With tenders amounting close to 2 trillion euros annually in the EU, it is critical that this market operates fairly and efficiently, supporting competitiveness and accountability. Data-driven insights can help make this happen, supporting buyers and suppliers alike in their procurement decisions
The skills needed to build knowledge graphs are precious, and so is sharing the knowledge on knowledge graphs. Chris Mungall continues his series on tips on building ontologies, ranging from the obvious need to clearly document them, to techniques for building them in a modular, normalized way.

OntoTip: Learn the Rector Normalization technique
The 2003 paper Modularisation of Domain Ontologies Implemented in Description Logics and related formalisms including OWL lays out in very clear terms a simple methodology for building and maintaining compositional ontologies in a modular and maintainable fashion. Anyone involved with the authoring of ontologies should read this paper, and strive to build modular, normalized ontologies from the outset
More tips: what are your options for serializing RDF? A lot, so Joep Meindertsma from Ontola tries to answer the question: What’s the best RDF serialization format?

What’s the best RDF serialization format?
Contrary to some other datamodels, RDF is not bound by a single serializiation format. Triple statements (the data atoms of RDF) can be serialized in many ways, which leaves developers with a possibly tough decision: how should I serialize my linked data?
More RDF, more releases. CSV2RDF is a streaming, transforming, SPARQL-based CSV to RDF converter. Version 2.0 has just been released, now with named arguments and a Docker image.
SANSA (Semantic ANalytics StAck) is a framework for large-scale analysis, inference, and querying of knowledge graphs. Version 0.6 has just been released, including features such as tensor representation of RDF and RDF data quality assessment methods.
Last but not least, RMLStreamer is a framework that executes RML rules to generate high quality Linked Data from multiple originally (semi-)structured data sources in a streaming way
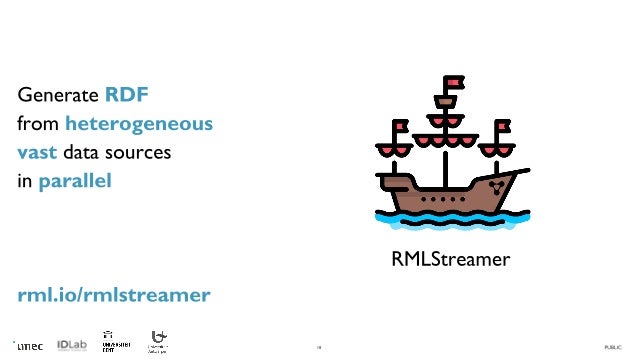
Parallel RDF generation of heterogeneous Big Data sources
We developed the RMLStreamer, a generator that parallelizes the ingestion and mapping tasks of RDF generation across multiple instances. RMLStreamer ingests data at 50% faster rate than existing generators through parallel ingestion
Most people are using one of the 2 more popular ways to model graphs, RDF and property graphs. RDF* is a proposal for extending RDF in a way that can facilitate mapping between them. One of the differences between RDF and property graphs are their semantics, so Chris Mungall explores a way to map RDF semantics to RDF*.
Olaf Hartig, a researcher from Linköping University who has proposed RDF*, takes a step further by providing a theoretical foundation for converting property graphs into RDF* data and for querying them using the SPARQL* query language.
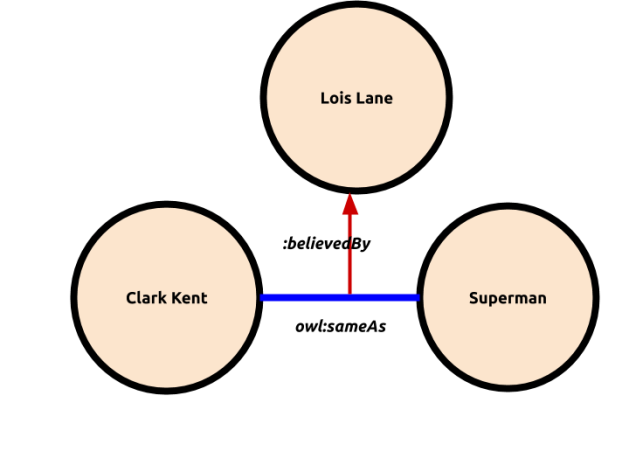
Proposed strategy for semantics in RDF* and Property Graphs
RDF* (and its accompanying query language SPARQL*) is an attempt to bring Property Graphs into RDF, thus thus providing an answer for differences in underlying datamodel and standardization of Property Graphs. But what about semantics?
Bob DuCharme is a well-known SPARQL expert. Here he explores Querying geospatial data from OpenStreetMaps with SPARQL.

Querying geospatial data with SPARQL
OpenStreetMap is a crowd-sourced online map that has made tremendous achievements in its role as the Wikipedia of geospatial data. OSM provides a SPARQL endpoint and a nice page of example queries. With their endpoint, you can list the names and addresses of all the museums in New York City (or, in RDF terms, everything with an osmt:addr:city value of “New York” and an osmt:tourism value of “museum”)